Animal brains use less data and energy compared to current deep neural networks running on Graphics Processing Units (GPUs). This makes it hard to develop tiny autonomous drones, which are too small and light for heavy hardware and big batteries. Recently, the emergence of neuromorphic processors that mimic how brains function has made it possible for researchers from Delft University of Technology to develop a drone that uses neuromorphic vision and control for autonomous flight.
The results are very promising: during flight the drone’s deep neural network processes data up to 64 times faster and consumes three times less energy than when running on a GPU. Further developments of this technology may enable the leap for drones to become as small, agile, and smart as flying insects or birds.
Abstract
Biological sensing and processing is asynchronous and sparse, leading to low-latency and energy-efficient perception and action. In robotics, neuromorphic hardware for event-based vision and spiking neural networks promises to exhibit similar characteristics. However, robotic implementations have been limited to basic tasks with low-dimensional sensory inputs and motor actions because of the restricted network size in current embedded neuromorphic processors and the difficulties of training spiking neural networks.
Here, we present a fully neuromorphic vision-to-control pipeline for controlling a flying drone. Specifically, we trained a spiking neural network that accepts raw event-based camera data and outputs low-level control actions for performing autonomous vision-based flight. The vision part of the network, consisting of five layers and 28,800 neurons, maps incoming raw events to ego-motion estimates and was trained with self-supervised learning on real event data. The control part consists of a single decoding layer and was learned with an evolutionary algorithm in a drone simulator.
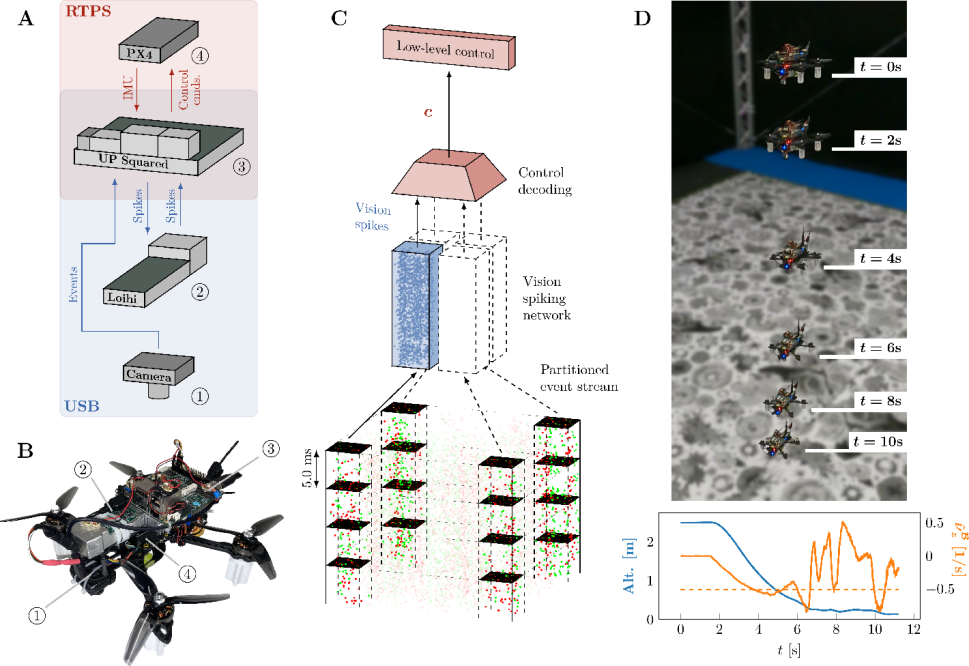
Overview of the proposed system. (A) Quadrotor used in this work (total weight 1.0 kg, tip-to-tip diameter 35 cm). (B) Hardware overview showing the communication between event-camera, neuromorphic processor, single-board computer and flight controller (C) Pipeline overview showing events as input, processing by the vision network and decoding into a control command. (D) Demonstration of the system for an optical flow divergence landing
Robotic experiments show a successful sim-to-real transfer of the fully learned neuromorphic pipeline. The drone could accurately control its ego-motion, allowing for hovering, landing, and maneuvering sideways—even while yawing at the same time.
The neuromorphic pipeline runs on board on Intel’s Loihi neuromorphic processor with an execution frequency of 200 hertz, consuming 0.94 watt of idle power and a mere additional 7 to 12 milliwatts when running the network. These results illustrate the potential of neuromorphic sensing and processing for enabling insect-sized intelligent robots.
A series of supplementary videos is available on YouTube here.
The full 18-page Paper is available here.
Source: ScienceRobotics